
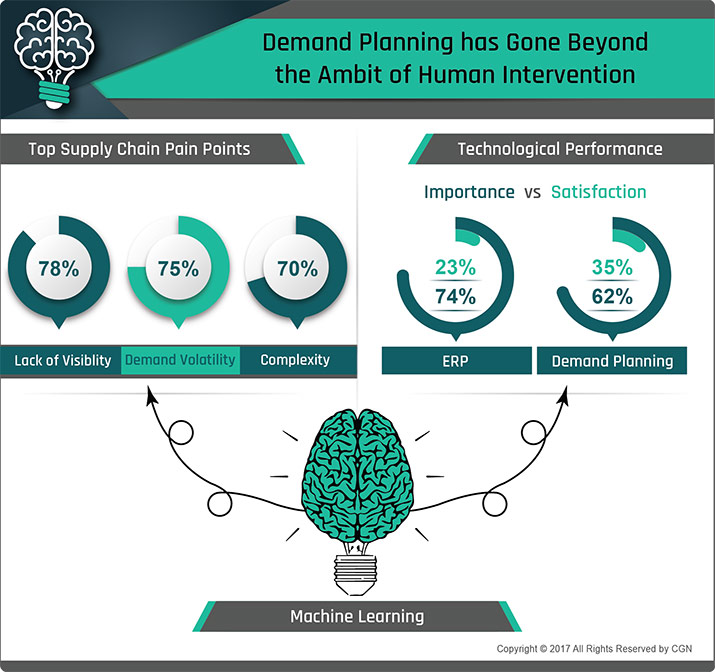
My discussion with clients on “Improving performance of demand planning function” always involved a lot of emotions from the clients. These emotions were not milder ones but extreme ones, consisting of hopelessness and anger. One client quoted it saying “Improving the performance of demand planning function is like trying to capture a soft sand with a fist." No matter how hard, a demand planner tries to capture market variability, the problems of supply chain visibility, demand volatility, and multi-channel supply chains belittle his efforts.
Challenges
In past two decades, companies had spent a lot of time and effort on technologies and processes to improve the performance of the demand planning function but the success was mostly elusive. The technology mostly worked on user defined algorithms which hardly moved beyond historical time series. The algorithms also ignored various external and internal factors like promotions, new product introduction, long-tail demand, demand variation across multiple channels and extreme seasonality while fore casting the demand. Thus, the forecasted demand varied a lot from actual demand and companies ended up with a lot of inventories.
On process front, companies collaborated among their various departments to get the right picture of the demand for quickly capturing the sensitivity. The collaborative process was slow and influence d by individual error and biases. Also, most of the inputs used to come to demand planners, at the last minute. Demand planners had to stitch those last-minute inputs with technological output to some how deliver some meaningful insights for a period. This process was inefficient. The result was instead of generating an accurate forecast for a period, the demand planners ended up with the manipulation of forecasted output.
Machine learning: The savior
The machine l earning technology can address the loopholes of existing technologies and inefficient processes. It does not follow the traditional approach, based on pre-defined and coded time series algorithm to predict the demand but learns from the fed data. It is capable of capturing only relevant factors while discarding the others. The machine learning technology is also sensitive towards the external and internal factors that change over time and adapt its outcome accordingly. It doe s not have to rely on collaboration with other departments as it is fed with actual data on fields. It is also able to capture the stimuli such a promotions and advertising across various demand channels to predict demand during that period. Implementation of machine learning technology doe s not require large investment; it can work in synchronization with the existing information management system.
As systems are getting more and more sophisticated, large & varied amount of data is generated across the supply chain and non-supply chain (social media) network. The companies must switch to machine learning to predict the realistic and reliable futuristic demand. The faster the companies adapt it, the greater competitive advantage they will have.
References
http://www.supplychaindive.com/news/Merck-KGaA-AI-machine-learning-demand-planning/432885/
http://www.supplychainquarterly.com/topics/Technology/20141230-machine-learning-a-new-tool-for-better-forecasting/
http://www.supplychain247.com/article/a_practitioners_guide_to_demand_planning
What we found interesting
"Here is an interesting video on the role of demand planning in improving supply chain performance:"